NIKHIL VADLAMUDI, Data Science & Business Planning Manager, AMD
How Machine Learning Is Improving Semiconductor Operations Semiconductor manufacturing is a high-stakes industry where efficiency, precision, and agility directly impact profitability. In recent years, machine learning (ML) techniques have emerged as tools to optimize operational decisions in chip production. Semiconductor engineers, operations managers, and tech executives are using ML to improve supply chain forecasts, streamline inventory, minimize equipment downtime, and maximize yield in fabs. This article explores how ML is applied in four key operational domains – demand forecasting, inventory management, equipment maintenance, and yield optimization – blending technical detail with strategic business insights. Real-world case studies and best practices illustrate the substantial gains achievable today and point to the future of smarter semiconductor manufacturing.
Enhancing demand forecasting for chip production
Accurate demand forecasting is critical for semiconductor production planning. Even small errors can cascade into costly overproduction or missed sales due to shortages. Traditional forecasting methods often rely on historical sales and human judgment, which struggle to account for the volatile and complex drivers of chip demand. ML offers a data-driven upgrade: algorithms can ingest a wide range of historical and real-time inputs, orders, economic indicators, market trends, customer inventory levels, and detect non-obvious patterns to predict future demand more accurately [1].
Leading firms are finding that incremental improvements in forecast accuracy yield major dividends. In one case study, NXP Semiconductors determined that its long-range forecast accuracy had stagnated around 70% for years; each additional percentage point of accuracy would reduce one full day of inventory, translating to millions of dollars saved annually [2]. By deploying ML models (e.g., neural networks and random forests) to incorporate factors like distributor sell-through and macroeconomic data, NXP was able to improve demand prediction performance. Internal trials demonstrated that these ML techniques could outperform the company’s legacy statistical models, potentially improving the average forecast precision by more than 20% [2]. Similarly, AI-driven forecasting systems can continuously learn from new data, adjusting to market shifts (such as sudden booms in automotive or AI accelerator demand) far faster than manual methods (FIGURE 1).
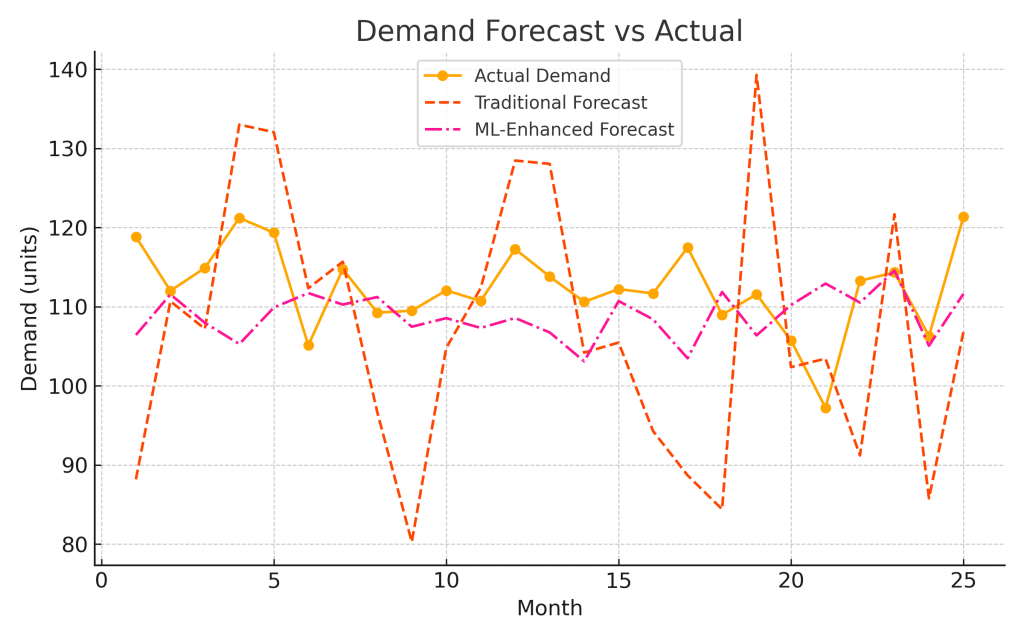
Real-world results underscore the strategic value of better forecasts. Incremental accuracy gains prevent chip shortages and excess stock, directly protecting revenue. For example, during the highly volatile 2020–2022 period, companies with AI-assisted forecasting were able to anticipate demand surges and allocate production slots more effectively, mitigating the impact of global chip shortage [3]. Best practices are emerging from these successes:
- Integrate diverse data sources: Incorporate not just historical sales, but also indicators like OEM production plans, economic indexes, and even alternative data (e.g., web search trends for electronics) to enrich training data [1].
- Continuously retrain models: Semiconductor demand can change with technology cycles and external shocks. High-performing companies frequently retrain ML forecasting models with the latest data to capture new demand signals (such as a sudden spike in electric vehicle orders) [1].
- Human oversight and domain knowledge: While ML finds hidden patterns, human experts provide sanity checks and scenario analysis. The most effective forecasting solutions push ML-generated predictions to planners, who can adjust for qualitative factors and then feed corrections back into the learning loop [1].
By blending ML algorithms with the expertise of supply chain analysts, semiconductor firms can achieve more agile and accurate demand forecasts. This, in turn, feeds into production planning and capacity decisions, helping to avoid both under-supply and oversupply scenarios (FIGURE 2).
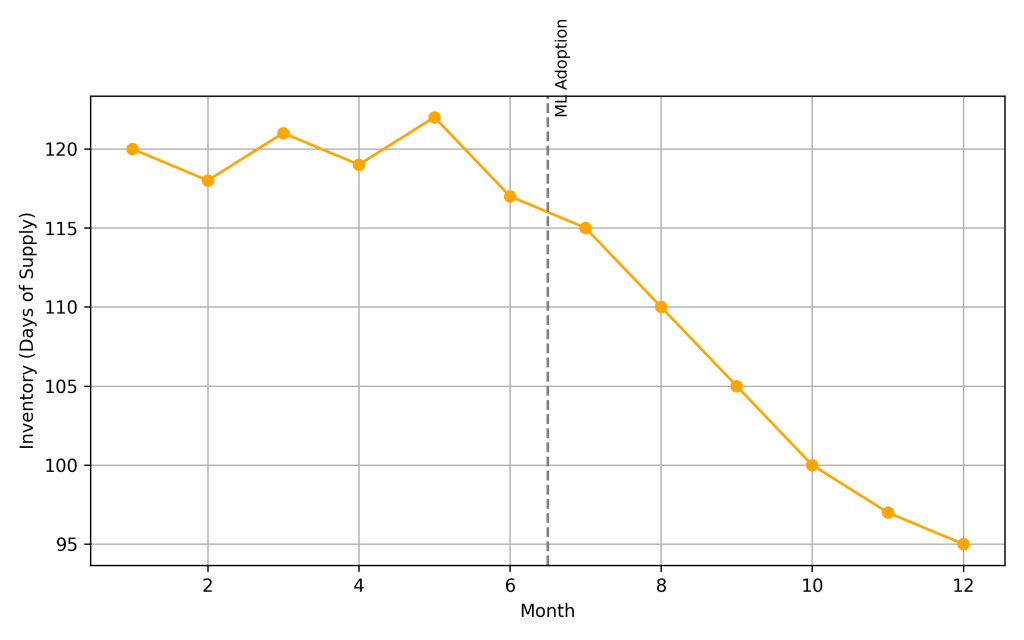
Improving inventory management via real-time optimization
Closely tied to demand forecasting is inventory management – another area where ML is driving significant improvements. Semiconductor supply chains are long and complex, so maintaining optimal inventory is challenging but vital. Excess inventory holds precious working capital and risks obsolescence, while insufficient stock of raw wafers, substrates, or spare parts can halt production lines. ML-powered analytics address this by enabling Realtime dynamic optimization of inventory levels throughout the supply network [3,4]. Traditionally, inventory targets were set by static models and manually adjusted periodically. Now, AI-driven inventory optimization systems continuously analyze consumption patterns, lead times, and even supplier risk factors to fine-tune buffers. For example, models can predict demand and variability for each component and automatically determine the minimum safety stock needed to ensure a given service level [5]. According to industry reports, adopting ML in inventory planning can reduce buffer stocks substantially – one AI implementation by a leading semiconductor manufacturer suggested a 15–20% reduction in required inventory, translating into 500millionto1 billion in freed working capital across the industry [6].
Click here to read the full article in Semiconductor Digest magazine.